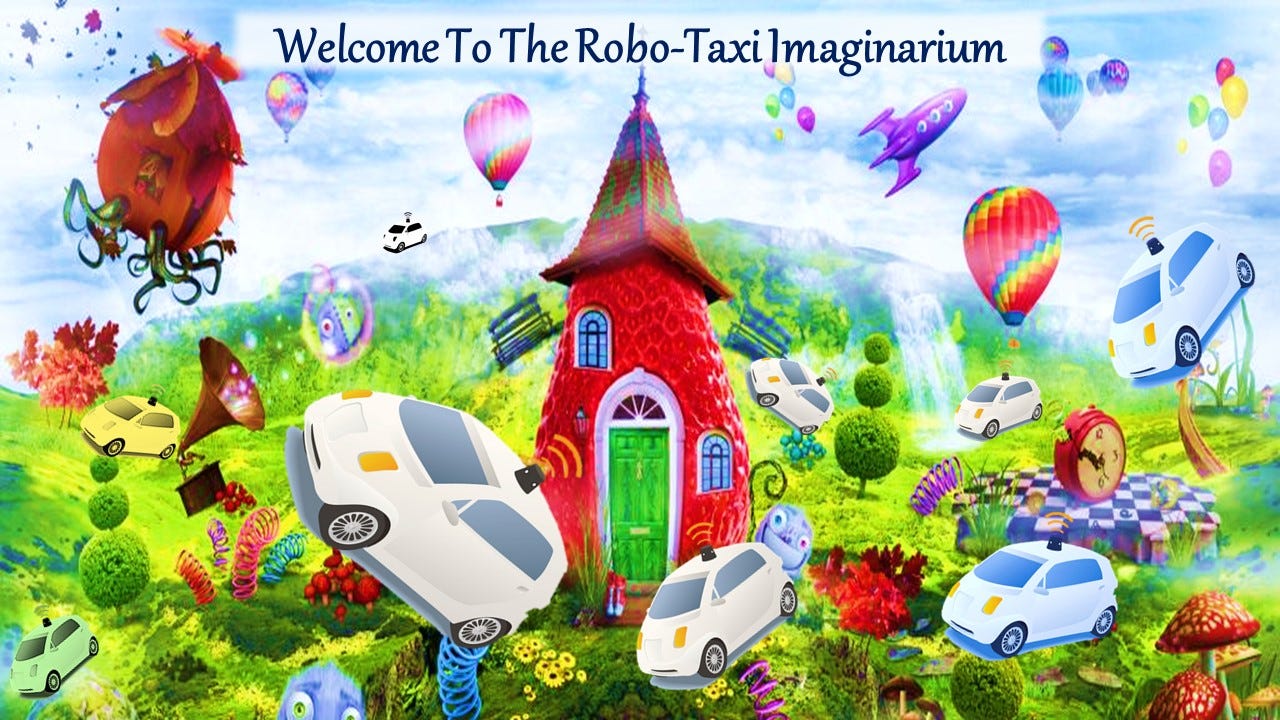
Driverless taxis were supposed to be here in 2020 - every major news headline told us so in the preceding few years. Between 2016 and 2019, venture capitalists furiously channeled billions into urban robo-taxis because they saw autonomous shared mobility as a multi-trillion-dollar industry by 2025. Governments were not left far behind either and some set out millions in grant funding for driverless taxi trials in their big cities in the hope of solving the urban transport challenges. Founders of driverless car start-ups emerged as the new crop of tech celebrities. There were conference circuits and big publications hyping up the robo-taxi revolution and ministers wooing and interviewing academic founders of self-driving car companies. It was a foregone conclusion that none of us were ever going to need our own personal car in the future because soon all vehicles would be connected, autonomous, and shared. The idea of an ‘Autonomous Uber’ had taken root.
Challenging this larger than life proposition at the time was very difficult when companies like Waymo were leading the charge on this use case. Tesla’s CEO declared in 2019 that Teslas would soon be full self-driving, making it possible for Tesla owners to earn money by sharing their cars on the Tesla network – an ‘appreciating asset’. Amidst this frenzy nobody cared to actually examine the use case economics or its unique technology challenges.
In this article, I will share a type of teardown analysis of the technical and commercial market-readiness of a robo-taxi service, because it has to-date and continues to represent the pinnacle of expectations linked to automated driving technologies.
The robo-taxi equation purveyed by industry analysts, investors, robo-taxi start-ups, and such like, in its most puerile form could look like this;
f (yummy, no brainer) = (Uber type scale and growth) X (Human Driver does not need to be paid) X (24/7 service) X (Vast data opportunity)
= $trillions in profits
The above equation has proven to be hard to solve. Expectations are getting reset closer to reality. Waymo’s valuation has been slashed, Tesla’s full self-driving is not yet here, late stage pivots away from Robotaxis have happened, and therefore many others are finding it an opportune time to throw in the towel on this use case failing at the technology front. Accompanying sound-bites such as; ‘so many more billions will be needed for this technology’, ‘regulation is not yet ready’, ‘autonomous fleets will be hard to manage’ etc. save face but can’t soothe investor anguish. After $18 billion invested to-date, the needle on robo-taxis has moved by naught.
Just to distinguish robo-taxis from other forms of urban mobility such as fixed route shuttles, I mean a commercial service capability that is based on a large fleet of passenger vehicles, that can:
1) be driven fully autonomously without a driver
2) pick and drop passengers from anywhere to everywhere, i.e. provide service without constraints of a limited zone of operation just as normal human-driven taxis do
3) operate day and night in most weather or lighting conditions
4) be at least as safe as a human taxi-driver
5) be cost comparable to human-driven taxi services available today
Let’s first examine the commercial hurdle rate before we look at the technical challenges of this particular urban mobility use case.
Commercial Barriers
First a bit about taxis – you get them almost everywhere, we can book them or hail them by the roadside, though Uber has shown a new level of user friendliness in booking a cab and paying for your ride. Taxis can pick you up from wherever you are and take you where you want to go. You can have a private or in some cases a shared ride. Regulated taxi services are expensive for all sorts of genuine reasons but then again, Uber has pushed the price down to a level where nobody actually makes any money, including Uber itself.
Uber type services have not unlocked any pent-up transportation demand but rather only shifted the demand from regulated taxis to a cheaper network alternative. Secular transportation demand is linked to growth in number of households and economic activity in relation to geographic clusters – these are smooth variables over the long term, not short-term trends that move due to changes in service pricing.
Many analysts, investors and technologists are quick to claim that robo-taxis will deliver massive economic returns by eliminating the cost of a human driver and running a 24/7 service with a robot-driver that doesn’t need to take any breaks (see equation above). In fact, far too many simplistic assumptions about operating a robo-taxi service fleet have been made and this is where the calculus begins to diverge drastically from reality.
There are three key action pillars to operating a robo-taxi service. These are (1) maintaining a sensor-laden fleet of robot cars, (2), operating a taxi service without any drivers in the car, and (3) creating a safe and informative in-cabin rider experience.
Robo-taxi Vehicle Maintenance
At the end of an operational service cycle (12 hours, 24 hours etc.), the driverless car in the fleet will need to be serviced for cleaning, re-charging (assuming electric cars) and diagnostics on the day’s performance. All sensors on the vehicle including cameras, RADAR, and LIDAR may need to be re-calibrated on at least a weekly basis. This could be a tedious procedure typically requiring expensive calibration-rigs setting the service operator back several thousand dollars a-piece. A self-driving taxi may produce up to 20Tb of data in a full day of operations which must be downloaded, catalogued and stored – this would be important for insurance and incident analysis. Downloading this data would easily take a few hours per taxi. If there are any repairs required to the vehicle such repairs could be carried out perhaps whilst data is being downloaded but before sensors are re-calibrated. Streamlining these operations would require a large and well-trained technical staff of vehicle engineers, perhaps 3 per vehicle, working in parallel much like a pit crew for racing cars.
Robo-taxi Service Operation
To operate a robo-taxi service first requires establishing safe pick-up and drop-off points for passengers. Human taxi drivers today are very good at this task. They are able to easily pull onto the side-walk, put on their hazard lights and stop on the side of a road and find a safe place to temporarily park to drop off and pick up passengers – but this won’t be possible for autonomous cars. For Robo-taxis, pre-determined pick-up and drop off points would need to be established. A streamlined communication channel would need to be set up so that the vehicle could notify passengers of its location and to ensure that passengers actually find their robo-taxi. (In a human driven cab, the cabby and the passenger both make the effort to locate each other, even after coordinating over a text message). A real-time autonomous fleet management system would also be required to monitor the state of each vehicle in real time, and this too is no small feat. It would not be enough to simply know where the vehicles are at any given point, but also how much battery range they have left, whether they are free or occupied, how many passengers are being carried, where each passenger is going, in addition to knowing the real-time state of the vehicle’s automated driving system. To manage all of this would require a large back-end fleet-technology and data management infrastructure requiring continuous engineering support staff for system management and maintenance. Tele-operation, for remote management of robo-taxis in need of sporadic remote assistance would add further cost and complexity, and over-the-air (OTA) bandwidth issues would need to be tackled for ensuring safe operation at all times without suffering OTA latency. Cybersecurity would also have to be addressed robustly in such a context.
Robo-taxi In-Cabin Experience Management
The in-cabin passenger experience will play a critical role in the comfort and safety of the rider, and would ultimately underpin the consumer adoption of robo-taxi services.It would be essential to have in-cabin remote monitoring to provide assistance to those who are unfamiliar with the service, or who have questions about the journey, or decide to change their destination at the last minute, or other very human things passengers do which taxi drivers easily accommodate. Then there would be the strange stuff (a long-tail of strange scenarios) – which could be a list of things that can happen within a driverless taxi service which no one can predict today. For example, what happens if an over-enthusiastic passenger jumps into the driver seat and tries to interfere with or disable the robo-taxi’s operation? What happens if a passenger has a medical emergency during their journey? What happens if a passenger tries to commit a crime in a self-driving taxi? Etcetera, etcetera.
To be safe and to keep things in check, an autonomous taxi service would need a large customer services staff to support and monitor in-cabin passenger requests and to bring about interventions requested by or required by passengers. This could be estimated at perhaps one staff member for every three robo-taxis to monitor and provide assistance to the passengers during a ride, but again 24/7 (therefore in reality 3 persons if there are 3 such shifts in 24 hours).
Back-of-the-Envelope Calculations
Given the above requirements for vehicles maintenance, service operations and in-cabin experience management, assuming a robo-taxi fleet of 20,000, the operation might require around 5,000 vehicle engineers working in shifts around the clock, and around 7,000 full-time customer services support staff. If we consider $10/hr as base pay for customer services support and $100/hr for vehicle engineers putting in 5hrs of work per day, the approximate staffing cost for the fleet comes to:
Customer Services: 7,000 staff x 24hrs/day x $10/hr = $1.68MM per day
Engineering Support: 5,000 staff x 5hrs/day x $100/hr = $2.5MM per day
Let’s assume that offline fleet operations staff are excluded from this equation and the entire fleet management is completely automated – this is already a big ask, but for arguments sake if we ignore this among many other things, a simple total for essential staff costs comes to:
Staffing Cost Per Day: $4.18MM (excluding all other engineering, support and management staff costs)
Now let’s look at one-time capital expenses for equipping a fleet of robo-taxis. Depending on your choice of car (Jaguar I-Pace, Toyota Prius), a single vehicle with necessary electronics for drive-by-wire control (a system to allow a computer to actuate the accelerator/brake pedals and turn the steering wheel) could set you back between $45k - $80K for a bulk order price.
So, on the lower end, the cost of vehicles would be:
Vehicle Cost = $45,000 per car x 20,000 cars = $900MM (nearly a billion dollars)
Now let’s look at another big component of capital expenditure – sensors. Robo-taxis will need a large array of sensors for adequate field-of-view coverage around the vehicle in 360 degrees, and sensor redundancy to ensure vehicles can operate at night time and in adverse weather – this means you will definitely need LIDAR (otherwise you won’t have enough sensor redundancy to run your fleet in very low-light conditions or when camera vision degrades).
Some robo-taxi prototype vehicles have more than 30 sensors – and this seems to be about the norm. For a 20,000-unit order, you could expect sensors, sensor mounts, wiring, computer systems, in-vehicle data storage, and mechanical/electrical installation to cost no less than $50K per car.
So, the other one-time capital costs per vehicle add up to:
Sensors/Electronics/Compute Cost = $50,000 per car x 20,000 cars = $1Billion
Therefore, to regroup, CAPEX for a fleet of 20,000 robo-taxi fleet would be $1.9Billion (assuming a very lax 10-year useful life) with direct staffing OPEX of around $4MM per day, which is equivalent to $1.2Billion per year assuming 300 days of operation per calendar year. The 10-year direct cost of a robo-taxi fleet would come to:
($1.9Billion upfront CAPEX) + ($1.2Billion OPEX per year x 10 years) = $13.9Billion
This excludes the CAPEX for backend, engineering development and going staff costs of the rest of the organisation including direct and indirect overheads and costs of capital.
But let’s say you secure $14Billion to build and operate a robo-taxi fleet (and basing this on the assumption that you actually possess commercial-ready urban full self-driving technology), let’s calculate how much could you expect to make, and when could you breakeven (assuming no other costs for the sake of simplification).
I have assumed a super-generous useful vehicle life of 10 years, therefore in order to recover just the $14Billion, the robo-taxi fleet would obviously need to bring in $1.4Billion each year.
Let’s assume that a single passenger journey costs $10 on average – this would be a fair assumption to work with and it entails that annually the robo-taxi fleet would have to deliver at least 140MM passenger rides. Assuming the aforementioned 300 days of operation each year the number of daily passenger trips for the fleet would be equal to:
Number of passenger trips per day = 140MM trips per year / 300 days per year = 466,667 trips per day.
For a fleet of 20,000 robo-taxis, this implies 23 passenger trips requirement daily over a 20-hour work day (466,667 trips/20,000 robo-taxis).
According to New York City Taxi and Limousine Commission – Morgan Stanley Research (April 2016), NY Taxis made 13 passenger trips per day. The average Uber managed 6 passenger trips per day, whereas Lyft and Gett clocked in at 3 per day, though Via got up to an average 15 passenger trips per day. Comparing this to our figure of 23 trips required per day for a robot-taxi shows that the likelihood of recovering the $14 billion would be barely feasible but only if demand for taxi rides was almost double of what it is today.
So yes, if everything went perfectly, and all self-driving technology challenges had been overcome and one were able to manage a highly efficient and streamlined robo-taxi fleet operation for 10 years – one could potentially recover $14 billion in 10 years if over this period taxi demand really surged(even though money has virtually no time value associated with it these days, would it be an ok thing to invest $14 billion of investment capital based on an imaginary demand surge expectation?).
It is important to note that in the above back-of-the-envelope calculation, I have not accounted for the following things at all:
▪ Tele-operation and back-end infrastructure CAPEX and OPEX
▪ Insurance costs, fleet or third-party liability
▪ Critical event response preparedness and operational safety costs
▪ Facilities for staff and vehicles (repair and maintenance)
▪ Management, marketing, legal or administrative costs
▪ Inflation adjustment over a 10-year period
Most importantly, I have not accounted for a single dollar in profit to the investment! In reality therefore, the robo-taxi equation is a bit more like this;
f (robo-taxi reality) = ($billions for self-driving tech development) X ($billions in CAPEX and OPEX) X (secular demand < 0.5 of what it needs to be)
= $billions in losses
I think you get the picture. Before I wrap up, let’s quickly examine a few technical challenges relating to the development of full self-driving technology for robo-taxis.
Technology Barriers
For an automated driving system to drive competently, it has to perform two essential functions; perception and mapping/localisation. Perception means the driving system must correctly process raw sensor data from on-vehicle sensors to develop an understanding of important objects in the driving scene – e.g. location of objects, identification of lane markings, classification of object types etc.
Localisation means the system must know its correct geo-position-location down to a few centimetres, in a globally consistent manner, all the time, as it drives. Localisation is done with the aid of a prior 3D High Definition (HD) environment map which encodes the centimetre accurate information about roadside infrastructure and static scene elements. The 3D HD map allows an automated vehicle to cross reference its sensor observations with respect to landmarks within the 3D HD map, to precisely triangulate its positions – in effect, 3D HD maps act like virtual rails for automated vehicles to drive along, and landmarks within the 3D HD map are a type of scene memory used by the vehicle to figure out where it is located in the real world with respect to the map.
Any error in perception or localisation is not acceptable from a safety standpoint – imagine the consequences of failing to correctly detect an object, or thinking you’re driving centred within your lane when you may be actually drifting into a neighbouring lane. Perception and localisation remain unsolved technical challenges by the robo-taxi enthusiasts, despite what you might read or hear. I won’t delve into the nature of the perception and mapping problems here, as I have written about these in my previous articles in details, but briefly, the state-of-the-art is as follows.
The perception nut is being cracked with neural networks
Neural networks are being trained furiously over the past 5 years with increasing levels of computational resources and ever-expanding datasets to help the automated vehicles recognise different types of objects in a driving scene.
You might ask the question: What is the problem with neural networks?
Answer:
It is impossible to construct an adequately representative dataset of every conceivable object an automated vehicle could expect to encounter, leading to a long-tail of edge-case scenarios which neural network perception systems are simply ill-equipped to handle. Objects of the same underlying type, e.g. a vehicle, may appear different due to size, orientation, lighting conditions etc., and the possible list of objects (not only road users) in an urban diving scene is indefinitely long. The world is large, complex, and ever-changing. This is an open-ended problem as object appearances could continually change over time – think about the difficulty in detecting newer concept cars with sporty swooshes and large windscreens, or new traffic lights installed as part of infrastructure upgrades. If you are interested, do have a read of my article ‘AI in Self-Driving – “It the Cake” ’ available at https://selfdriving.substack.com/p/ai-in-self-driving-it-the-cake
Building 3D HD maps is a non-convergent problem
In mathematics, a non-convergent series is a series for which an infinite sequence of the partial sums of the series does not have a finite limit.
You might ask the question: Why is building 3D HD maps, a non-convergent problem?
Answer:
Under most approaches, building 3D HD maps requires driving every road around the world multiple times in both directions with a fleet of mapping vehicles and human drivers to collect high resolution data for centimetre precise three-dimensional maps. This in itself is a tall order, and would require unimaginably large sums and effort over several years that robo-taxi fleets would have to undertake, or procure 3D HD maps from a third party. The more pressing problem with 3D HD maps is that under most approaches, even if these are constructed for global road networks, the challenge always remains in respect to their constant updates. As 3D HD maps capture very precise environment information, they could have a short half-life and become unusable and out-of-date for even minor environmental changes. For example, A 3D HD map encoding buildings would need to be updated when building facades change, a map encoding the location of foliage would need to be updated when seasons change, a map encoding the location of roadside infrastructure like bus stops or signs would need to be updated whenever these road features undergo maintenance or updates. Roadworks in most cases completely throw off a 3D HD map, and require the entire map to be reconstructed from scratch. This is where the challenge lies for 3D HD maps -accounting for all of the small environment changes that occur on a day-to-day basis in the real-world, ensuring that such changes are accurately reflected in the 3D HD map. If you are interested in a click down on this topic, do look up my article on prior maps for self-driving cars titled ‘When self-driving cars cannot self-drive’ explaining the mapping challenge in detail. It can be found at the link: https://selfdriving.substack.com/p/when-self-driving-cars-cannot-self
To sum it up therefore, the question I ask is;
In a scenario where the underlying self-driving technology for urban robo-taxis is nowhere in sight of being commercial-ready and an optimistic economic case for robo-taxis being so tentative and flawed, are investors just roaming about in the robotaxis imaginarium??