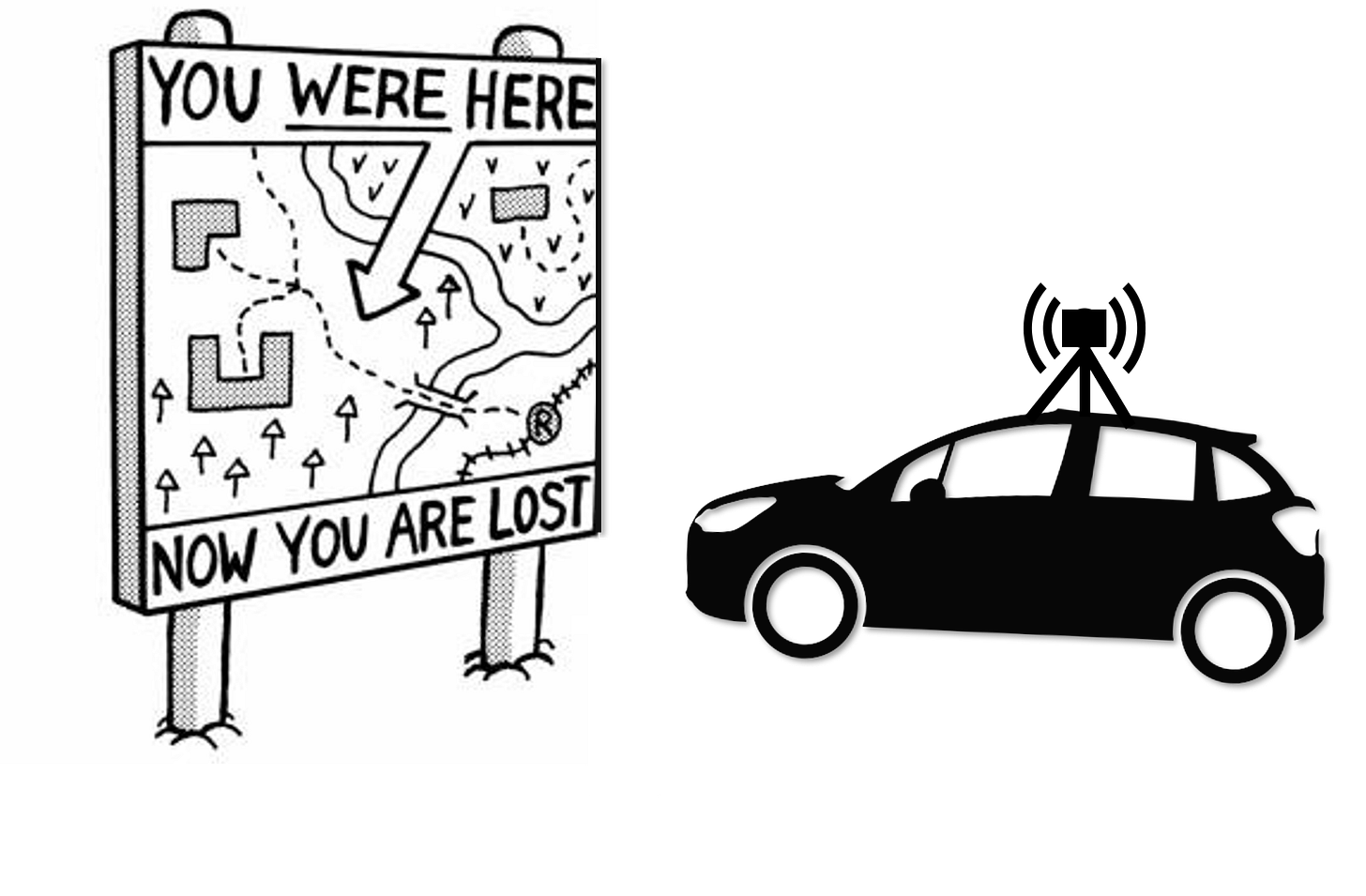
Self-driving cars depend on 3D High Definition (HD) maps to drive autonomously. HD maps, unlike common navigation maps we use everyday, do not exist and ordinarily HD maps need to be constructed through first manually pre-driving a route several times. This allows for data to be collected and collated from multiple pre-mapping runs. The harvested data can then be represented in the HD map as a high resolution 3D picture of the physical space and scene surroundings. The data collected in pre-driving requires various features of the route to be accurately annotated as part of the HD map building exercise; for example, lane markings, locations of junctions, locations of merges and splits upon road as well as several other static elements of the scene-surrounding with centimetre level precision. Various self-driving technology approaches adopt different sensors and methods for building HD maps.
A safety-critical aspect in adopting any particular HD prior map is that once created, it has to be kept updated with the same level of precision to account for changes that may have occurred anywhere along the pre-mapped route. Initiatives are currently underway around the world, hoping to successfully tackle the challenge of creating HD maps for autonomous vehicles, and research is exploring methods for maintaining these maps.
HD maps put a limit to where self-driving cars can drive autonomously. Until such time HD maps become available throughout the world, autonomous vehicles will remain feasible only on geo-fenced routes. This is currently the only viable option for most self-driving technology companies. Even global tech giants developing self-driving technologies have remained bounded within limited geographies and have not been able to scale for this reason among others.
Global map providers are striving to achieve a breakthrough to enable creation of globally scalable HD maps for self-driving. Besides the scale of the initial challenge confronting map providers – i.e. creating prior maps for each and every street around the world, a bigger challenge they face is how such maps could be kept precisely updated all of the time, all around the world. In view of the technological, financial, and procedural challenges to reach this goal, it becomes apparent that self-driving technologies will be unable to scale geographically until a fundamental breakthrough is achieved in mapping.
We started developing self-driving technology for the automotive sector in 2017 and we recognised the challenge of scaling HD mapping. We set out to tackle the challenge of building and scaling of HD maps from the very start, as a foundational capability of our automated driving software. Having now achieved substantial progress in both domains, I have decided to chronicle our journey and describe how we set out to resolve the dual challenge.
You may have come across the idea that HD maps for self-driving cars are a type of an ‘a-priori sensor’. Many map providers advance the idea that over time HD maps would act like an ‘operating system’ for self-driving cars by delivering a ‘safety supervisory’ function.
To better understand HD maps, let me first provide a review of exactly what role HD maps play in self-driving cars besides localization (a process of fixing the position of the self driving car with respect to the HD map by observing features in the driving scene). Self-driving cars need to accurately perceive all obstacles in a scene to avoid collisions. Self-driving cars must be able to distinguish between two broad categories of scene elements; static and dynamic. An element of this required information is meant to be aided by HD maps. A deeper examination of these two categories reveals that a simple two-category view confounds the role and capability of HD maps.
Examining the role of HD maps
HD maps encode fixed scene elements including fixed-static obstacles as opposed to dynamic elements that are ordinarily not encoded. The following discussion highlights the underlying issues in dealing with scene obstacles.
(1) Are dynamic obstacles always in a state of motion or can these be in a still state as well?
Self-driving cars need to interpret layers of information pertaining to each unique dynamic obstacle of relevance with the ultimate goal that a future path of any dynamic obstacle may be accurately predicted. Consider the fact that based on an obstacle being observed in a dynamic state, there would be no reason for a self-driving car to presume that such an obstacle will necessarily not ever come to be in a still state under certain conditions (for example, it must not be assumed that a moving car in front will always be moving).
Some dynamic obstacles may be of the nature that can inherently switch between a dynamic state and static state, e.g. a vehicle or a pedestrian. Other dynamic obstacles may be of the type that while being observed in a dynamic state, are likely to revert to a still state but not have an inherent capacity to effect a change in their state between being dynamic or being static, e.g. a shopping trolley that is observed while it is rolling away.
This nuance in defining what a static or a dynamic obstacle is, means that from the perspective of a self-driving car that seeks to predict the future path of an observed dynamic obstacle, it cannot treat all dynamic obstacles in the same way. This is because some dynamic obstacles can assume a dynamic or a still state and be inherently able to effect a change in their state, whereas other dynamic obstacles can assume a dynamic or a still state without inherently being able to effect a change in their state. Therefore, all dynamic obstacles are not the same with respect to how their future motion ought to be predicted.
With reference to the creation of HD maps, the general view is that dynamic obstacles are ordinarily understood to not be encoded in them regardless of whether such obstacles have come to be in a still state or remain in a dynamic state, and regardless also of whether an obstacle can inherently effect a change in its state or not.
There is no general expectation for HD maps to encode any dynamic obstacles that have come to be in a still state along the intended path of a self-driving car unexpectedly, e.g. a broken-down vehicle, an incapacitated pedestrian, abandoned shopping trolley etc. An automated vehicle has to rely on its real-time scene perception to safely deal with a broad range of unexpected (and therefore unpredictable) still-state obstacles along its path and no type of enablement from a prior HD map would be available. This means that a self-driving car needs to sense and perceive such types of unpredictable, still-state obstacles through its on-board sensing and perception technologies.
(2) How do static obstacles behave and how do HD maps deal with static obstacles?
It is ordinarily expected that static obstacles are encoded in HD maps. Let’s examine if the ordinarily held view is accurate, by probing into various types of static obstacles.
Consider first, a category of static elements such as those representing scene structures of a more permanent nature, e.g. road kerbs, highway gantries, tunnels/over-passes, exit ramps, dividing islands and signage boards etc. We can call them ‘permanent structures’.
A second category of static elements could be scene structures of a more transient nature, e.g. structures that are put up for a brief period of time as needed, e.g. traffic cones, Jersey barriers, temporary road-blocks etc. We can call them ‘emergent structures’.
A third category of static elements, create structure and comprise demarcations directly upon the road surface, e.g. cat-eyes, lane markings, other road markings, as well as signage elements along the road side. We can call them ‘road level structures’.
It would also seem appropriate to consider traffic lights as a fourth category of static elements, and this category may include other types of electronic signage as well. While it could be argued that most traffic lights should be classified as a permanent structure, temporary traffic signals would then not be accounted for in that category, since temporary traffic lights are an emergent structure. For convenience, one could therefore consider all traffic lights as a separate category of static elements and include all electronic traffic signage in this category as well. We call this category ‘electronic structures’.
Permanent structures are part of the road infrastructure. In view of the massive challenge of updating and maintaining HD maps, permanent structures are highly suitable for encoding into HD maps. This is because securely built-in structures are most resistant to change, and if a change does happen it is clearly in context of pre-planned, road infrastructure-level change. It should be reasonable to assume that in the long run, information regarding (pre-planned) road infrastructure-level changes could be encoded into HD maps on the basis of coordinating activities between map providers and authorities responsible for infrastructure-level road works. It maybe reasonable to assume that the HD map providers would re-survey such sections of roadways for ensuring that their HD maps remain updated. Nevertheless, the evolution of coordination mechanisms is subject to the road authorities and infrastructure developers becoming incentivised and willing to assume the requisite operational and administrative overheads for the task. From the perspective of HD map providers, the successful coordination of such activities worldwide may present a very high hurdle rate. However, it is quite plausible that HD map providers could be able to correctly encode and keep updated, permanent structures into their maps around the world.
Emergent structures in many instances represent temporary static elements that are required to be put in place as part of an emergency response mechanism at short notice due to unplanned events such as road accidents, vehicle breakdowns, or debris spillage from vehicles. In other instances, emergent structures may be put up as part of planned road works with variable lifespan of these transient structures. Emergent structures are a highly challenging area for HD map providers to encode, while being an extreme, safety-critical aspect for self-driving cars. Emergent structures, (e.g. traffic cones, Jersey barriers, road blocks) placed upon the drivable road surface in any context (planned or unplanned), produce such a high risk of catastrophic impact for the self-driving vehicle that assuming this type of risk on account of a deficient HD map is not an option. Currently, there is no viable method or mechanism for HD map providers to encode unplanned emergent structures into prior HD maps robustly and consistently, at a global scale. In a hyper-local context the HD map could certainly be created to encode the locations of traffic cones, however doing so in occasional instances in no way translates into a methodology for scalability everywhere.
In respect of planned road works, it would perhaps be expected that the placement of emergent structures, e.g. a 2-mile-long traffic cone row would be according to a prescribed guidance and a placement plan with respect to road-type. However, it remains highly questionable whether the centimetre-precise location of each individual traffic cone could also be prescribed in any such guidance or the plan. A related aspect would be to consider and evaluate if the precise positioning of each traffic cone deviates in implementation, even if according to a placement plan, as things could differ when traffic cones are actually placed on-site by the crew. An additional possibility further complicates the picture as certain emergent structures may move after placements, e.g. a number of cones may fall over or be nudged from their original position due to any reason.
In summary, the encoding of emergent structures is an open challenge for HD map providers, whereas it is an absolute safety-critical requirement for self-driving cars to be able to respond to each and every emergent structure, whether with or without assistance from any type of HD maps.
Road level structures, such as lanes (whether demarcated by lane markings or by cat’s-eyes) are the fundamental path delimiters for self-driving cars. Similarly, road level structures such as roadside signage, also deliver safety critical information, e.g. road speeds and road rules (e.g. no entry, right turn only, one-way flow etc.). Self-driving cars need to adhere to all rules by consuming this information delivered by all road structures. It may be fair to say that the centre-piece and the main delivery focus of HD map providers to self-driving cars has thus far been providing this particular category of information – i.e. the information that is otherwise delivered to human drivers through their sense of sight.
Lane markings are a key road level structure. It is clear that human drivers are easily able to detect lane markings, even when lane markings are in a highly degraded condition. On the other hand, the on-board perception technology of self-driving cars is considered to be fairly less competent than human beings in detecting lane markings when the markings are heavily degraded.
A self-driving car’s on-board perception for lane markings is commonly achieved through cameras via neural networks or classical computer vision for image analysis. The accuracy and robustness of lane perception from on-board cameras is variable under different technology approaches and may further be susceptible to factors such as; the presence of glare from vehicle head-lamps, road wetness, camera exposure changes while passing under bridges or emerging from them, excessive degradation of the lane markings themselves, ‘ghost lanes’ due to road works residuals, salt and pepper noise due the type of road tarmac, occlusion of lanes as in heavily congested traffic, reduced image capture horizon as on undulating roads, or occlusion of lane markings from snow, mud etc.
Even if we were to exclude the scenarios when road markings do not exist in the first place, a review of the various challenges in lane detection clearly presents why lane perception is considered a significant challenge for self-driving cars and that is why HD map providers are prioritizing this aspect of information.
Lane Centring Control (LCC) is a key component capability for automated driving, and lateral control is predicated on achieving LCC very accurately and robustly. It is therefore clear and logical that without highly accurate and robust lane perception, self-driving cars would not be able to achieve LCC. This capability becomes increasingly more safety-critical at the more advanced driving automation capabilities, e.g. ‘Autonomous Lane Change’ (ALC) manoeuvres.
The LCC and ALC challenge in highly automated driving is even more pronounced at higher driving speeds, while dealing with high road curvature, during night-time and in bad weather. Any lane perception limitations can severely degrade LCC and ALC.
Given the challenges of lane perception, the provisioning of centimetre-precise lane markings in HD maps is a useful redundancy to the on-board sensing and perception technology. Precisely encoded lane marking locations in the HD map could impart a very precise functionality as a redundancy for lateral control, if and when on-board sensing and perception technology is challenged in certain scenarios.
In theory, it is possible for HD maps to serve as a safety-critical redundancy for lane perception, but how does it sit in the context of the global scale of the problem? This implies that somehow, a method must be found by HD map providers to achieve the capturing, storage, retrieval and constant updating of the precise annotations of each and every lane marking on every single road around the world (both directions of travel) in order to deliver a globally scaled solution.
Achieving just this particular task at a global scale is a daunting challenge. Even for selected sections of roadways, HD map providers need to deploy very large fleets of manually driven vehicles or ADAS equipped manually driven vehicles in order to: 1) acquire data through manual driving, and; 2) annotate all of the lane markings in that data through some mechanism (whether manual or automated). In order for HD prior maps to provide a robust and persistent redundancy, it would be essential that the data acquisition through fleets is not just a one-time exercise but an on-going exercise. Furthermore, this also entails that the annotation task is also not a one-time activity but rather an on-going exercise, all over the world.
In order for HD maps to function as a back-up or fail-safe redundancy to lane perception, it would become relevant to consider two aspects concurrently: 1) the ‘Redundancy Persistence’ (RP) factor, which would be the duration of time during an autonomous drive, over which the HD map robustly provides lane level information, and 2) the ‘Mean Time Between Failure’ (MTBF) of the lane perception redundancy, as expressed in terms of ‘map breakage’ (which could be based on a number of collective ‘map failure states’ as well as the ‘localisation failure state’).
The MTBF of a prior map would be inversely proportional to its RP. Evidently, an HD map with a greater RP would be preferred to a map with a lower RP value. This attribute of an HD map would have to be first validated and then expressed by the HD map provider as a safety validation requirement before such an HD map can be used as a safety-critical redundancy.
The encoding of roadside signage into prior maps is yet another scaling challenge for map providers in context of road level structures. The verification of road level structures being accurately encoded in the HD map could be achieved on the basis of information from the infrastructure placement and maintenance authorities, however a standard, global, semantic ontology has yet to be developed by the HD map providers for expressing the large variety of road signage around the world, in order to make it commonly interpretable.
Electronic structures include traffic signals, temporary traffic lights and electronic signage on highway gantries. In the future, Infrastructure to Vehicle (I2V) communication could send electronic updates to self-driving cars. In the interim, it may be theorised that HD maps could encode the location of the permanent traffic signals as well as the location of gantries (on which electronic signs are present), for conveying an advanced opportunity for self-driving cars to be looking out for these electronic signs and interpreting them. In all cases, HD maps could really only go as far as providing the specific locations where electronic structures are installed whereas determining the ‘electronic signalling state’ of the electronic structures would be up to the on-board sensing and perception technology.
The above issue also applies to electronic variable speed limit signage on highway gantries. A prior map could only pre-inform a self-driving car that a gantry has electronic signalling systems installed upon it, however the on-board sensing and perception technology could not be assisted further by the HD map with respect to actually detecting and interpreting the ‘electronic signalling state’ of changeable signage on a gantry. That is why ‘Variable Speed Limits’ (VSL) determination is a challenge for self-driving on highways/motorways and HD maps can do little about this except encoding the locations of electronic structures.
(3) What else can HD maps enable for self-driving cars?
Location of merges on to highways: When travelling on highways, the pre-knowledge of the precise location of upcoming ‘merge-ons’ and ‘merge-offs’ is a very useful category of information for self-driving cars in advance of approach. The expectation of a ‘merge-on’ would enable a self-driving car to determine ahead of approach, a possible lane change manoeuvre in order to reduce risk from other traffic that would be merging onto a highway. Alternatively, a self-driving car could decide to perform anticipatory speed modulation and be on the specific ‘look-out’ for vehicles merging onto its ego-lane at an angular merge trajectory.
Location of merges off of highways: In the same way, information from an HD map of an up-coming ‘merge-off’ can be used to trigger certain decisions by the self-driving car. Close to ‘merge-offs’ a number of other vehicles that intend to take an exit from the highway, may begin changing lanes to the exit lane. The prior information regarding an up-coming ‘merge-off’ could assist a self-driving car to determine a lane change manoeuvre to a centre lane, thereby evasively moving out of a lane that is closest to the exit. Alternatively, a self-driving car could make a decision to proceed in a lane that would provide a transition to an exit lane, while proceeding straight if it intends to continue along its travel straight on the highway.
The degree of road curvature: Having curvature information of an approaching segment of road can provide ‘look-ahead’ cues to a self-driving car for modulating its speed in response to the degree of curvature. While this can be achieved directly based on any long range on-board sensing, HD maps are most suited to providing ‘look-ahead’ curvature information to self-driving cars. In highly adverse weather, as during a heavy rain storm, the range of on-board camera perception may become degraded and a backup redundancy of an HD map providing curvature information would be safety critical. Curvature information is used in advanced ADAS applications, such as determining ‘Closes In-Path Vehicle’ (CIPV) and adaptive headlight control.
Route navigation information: Satellite navigation systems (Satnav) guide human drivers in determining route goals. In multi-lane scenarios, Satnav provides lane choice guidance to human drivers, identifying which lane a vehicle must be ‘kept-in’, in order to adhere to the overall route goal. HD maps for self-driving cars can also encode such route information.
Summarising the roles of prior maps:
List of scene elements – generally encoded vs. generally not encoded in most HD maps
Dynamic Obstacles (dynamic state) - Not encoded
Dynamic Obstacles (expectedly still) - Not encoded
Dynamic Obstacles (unexpectedly still) - Not encoded
Static obstacles (unexpectedly dynamic) - Not encoded
Static obstacles (permanent structures) - Encoded
Static obstacles (emergent structures) - Not encoded
Static elements (road level structures) - Encoded
Static elements (electronic structures) - Location can be encoded/ signalling state not encoded
Merges & exits on highways - Present in the base navigation layer of existing navigation maps
Degree of road curvature - Encoded or can be derived from encoded data (accuracy unknown)
Route navigation - Present in the base navigation layer of existing navigation maps
When we carefully examine the role of HD maps for self-driving, it becomes clear that the central focus of HD map provisioning is on the encoding of above items 5, 7 and 10, whereas other items are either not encoded or are simply based on existing navigational maps. For many other items, the scale of the challenge is either too vast or procedurally too cumbersome or technologically unviable at present.
Certain essential items that are safety critical e.g. item 6 (emergent structures) are not encoded as it is an open challenge. Other items, e.g. item 8 (electronic structures) are more readily suited to being provisioned via I2V with the locations and precise ‘electronic signalling state’ which HD maps cannot do.
In light of the large number of key required enablements for self-driving that HD maps do not or cannot encode today, it becomes clear that HD maps cannot currently be readily viewed as ‘a-priori sensors’ but rather only as fairly limited attribute databases. We can only hope that the attribute scope, robustness, persistence and geographic scaling may be improved in the long run.
This analysis is an attempt to share with my readers, what the starting point of our journey was when we set out to develop a new ‘mapping technology’ for self-driving cars - one that does not require pre-driving any roads and self-updates road level features. Our analysis also guided the development of several aspects of required innovation for the most challenging aspects of ‘perception technology’ for self-driving cars and ADAS vehicles – the goal always being to develop technology that could overcome the scalability challenge for self-driving cars in a meaningful way.
We have since, successfully developed the underlying technologies ground up (which we continue to refine and test, as we deploy from UK to US), and we have also developed detailed metrics to measure and verify outcomes scientifically. If you are interested in reviewing metrics that can be applied to HD maps for self driving, you may enjoy reading Propelmee’s safety architecture ‘CARSAV’ (Comprehensive Architecture for Road Safety of Autonomous Vehicles) which can be downloaded from our website https://propelmee.com