AI in Self-driving - “It the Cake”
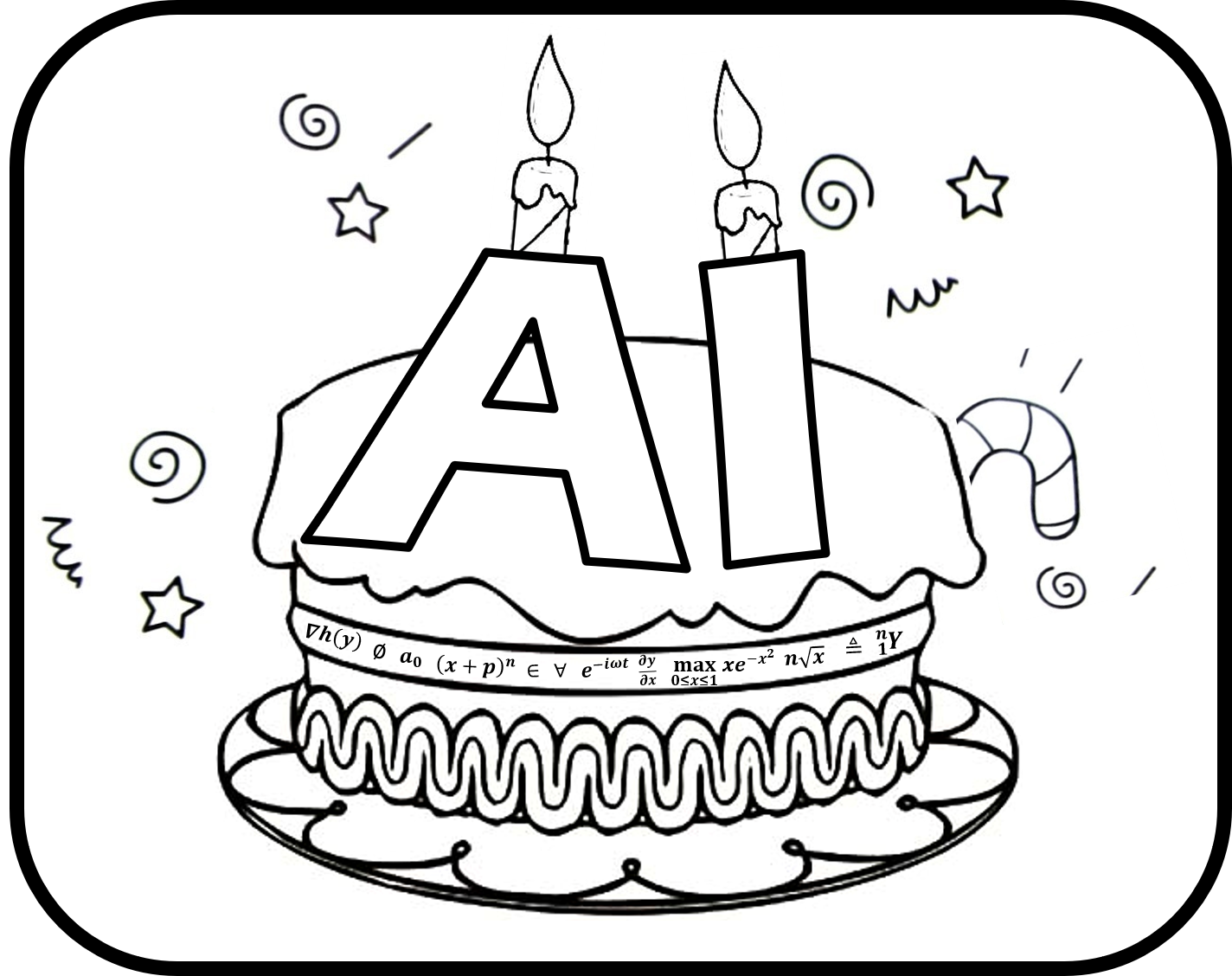
To ensure operational safety of self-driving technology, it is imperative that the technology must never fail in detecting all relevant obstacles in the driving scene. It must also never fail in correctly determining where the vehicle could drive. These are two central safety assurance requirements of the perception function of self-driving technology. The perception function bounded by these two core requirements is the very first guarantor of operational safety of a self-driving vehicle. Without robust perception that meets these two requirements all the time, in all scenarios of an Operational Design Domain (ODD), it is not possible to Verify & Validate (V&V) the self-driving system comprising downstream or parallel functions such as; localization, behavior-prediction, planning and control.
Each company developing self-driving technology claims that it has solved the perception challenge, and while the claims of some of them may be partly valid – there is very little clarity on how any third parties; e.g. consumers, regulators or insurers, could establish if these technology claims are actually valid or not. From the perspective of ensuring consumer safety, regulators around the world are left grappling with the question of whether or not perception has been solved by the industry as a whole. Regulators face an uncertainty of focus on this matter, not knowing for sure, if more of their scrutiny should be directed at ensuring safety of the self-driving technology as a system or whether perception deserves a special focus.
In context of academic research, it is generally viewed that benchmarking against prominent datasets suffices for establishing how well a researcher has done in solving a part of the perception challenge. When it gets to commercially deployed automated driving systems, the V&V issue becomes much more complex than what a simple benchmark score can provide for academic research purposes. Confounding factors such as; dataset bias, sensor configuration, algorithm run-time on deployed compute, generalisability across data and edge-case response, are some of the issues that take on much greater significance in comparison to pure academic research.
In relation to evaluating the quality of perception of a self-driving system, simulators are often put forth as a potential tool – despite clearly known limitations of simulators in terms of robust sensor models and behavior realism. Leading simulation developers are still working on resolving the open challenges for simulation, and none currently claim that their platforms today satisfy the V&V requirements for all perception approaches used in self-driving technology.
With some academic benchmarks and under development simulation platforms, regulators are constrained in establishing the state of technology-readiness of the perception function and can only rely on what is being stated by self-driving technology companies themselves. However, self-certification can only go so far, as commercial deployment at large scale needs independent regulatory oversight. A responsibility devolves upon all interested parties to present viable solutions for addressing the perception V&V challenge in self-driving.
In general, self-driving technology companies have comprehensive and well-established methodologies for exercising scrutiny over the safety of their perception technology. There is no reason why some of the insider knowledge cannot be shared with regulators, insurers and consumers. Quite naturally, the motivation would be low for self-driving tech companies to hand out a testing tool-kit to regulators in order that regulators may then apply such tool-kits to exercise scrutiny upon them, however, the safety imperative is forceful enough to at least start a meaningful conversation on the topic of assuring operational safety of perception.
Artificial Intelligence (AI) in Perception
Self-driving technologies rely on ‘Neural Networks’ to identify a new observation in the driving scene, by classifying it into a particular category of ‘obstacle type’ that the network has been ‘taught’ beforehand. This is achieved by showing the network several examples of what various different obstacles of a certain type look like. The network ‘learns’ the task of identifying a new observation as belonging to a particular class (type) by comparing and matching certain ‘features’ of the new observation, with the ‘characteristic features’ of other observations that it was shown during training as belonging to a particular class of obstacles. A prediction made by the network is compared with the ground truth and the network continues to learn on the basis of how accurate it is becoming in its predictions – but in reality this never happens, and many other issues still remain even when a network does become almost as good as the ground truth on the training dataset.
For example, one issue is that the specific ‘characteristic features’ that a neural network ends up learning are not controllable by the designer of the neural network. In this context, the designer of the neural network only determines what training dataset is to be used for training the neural network, i.e. the designer determines the variety and the quantum of data as training input to the neural network. Even so, the designer exercises no direct control over which characteristic features the neural network ends up learning. As a result, the designer can never determine with certainty, how accurately the network will make output ‘class determinations’ when the network encounters previously unseen data, i.e. data out there in the real world that is different to the training dataset used.
The output ‘class determination’ accuracy of a neural network is therefore evaluated on the basis of statistical measurements, by reviewing, how well or how poorly, the ‘trained network’ performs on various new data instances, and determining statistical performance parameters for the neural network in terms of: ‘true positives’, ‘false positives’, ‘true negatives’ and ‘false negatives’.
The ‘True Positives’ Showcase
Designers of neural networks know that just for purposes of producing an output showcase, they could fairly easily achieve a ‘good-looking’ performance of the network with respect to ‘true positives’ if they show their network running on the very same data that they used to ‘train it’ on. Moreover, a neural network may also achieve early good results on a dataset that is fairly similar to the training dataset used by its designer. However, regulators, insurers or consumers may not necessarily understand that it would be a risky thing to conclude that the performance quality of the same neural network would be as good as a ‘showcase’ shown to them by the designer when the network operates in real life, as deployed on a self-driving car – and this is where a critical gap exists.
On the other hand, AI experts know and understand that achieving a statistically measurable high performance, on previously unseen data, that is dissimilar to the training dataset employed for ‘training the neural network, remains an open technology challenge in the field of AI. A review of Table 4 from (BDD100K: A Diverse Driving Video Database with Scalable Annotation Tooling by Yu et.al - https://arxiv.org/pdf/1805.04687.pdf shows a dramatic domain shift in network performance when trained on one dataset and tested on another previously unseen dataset. Neural network performance on unseen data dropped by up to 37.2 percentage points for mean IoU score. Both datasets were extensive and covered a wide variety of driving scenarios and object types. For car detection, a performance drop of up to 63.3 percentage points for mean IoU score was observed when switching between datasets. This then takes us back to the original consideration I opened this article with - a self-driving car always being able to detect all relevant obstacles in the scene and always being able to detect and determine where it should drive. If a neural network is not highly performant on data it has not seen before, then it would naturally be inferred that such a network cannot perform the perception task robustly.
‘True-Negatives’
True negatives occur when a neural network correctly identifies the absence of an obstacle in the scene.
‘False-Positives’ and their impact
False-positives generated by a neural network clearly impact the operational safety of a self-driving system. If a network determines that something is present in the scene when in fact nothing is there, a very silly thing happens to a self-driving car – it applies brakes to slow down or stop for a phantom obstacle and often ends up getting rear-ended by a human driven vehicle. There is no way for a human driver to anticipate a sudden stop or hard braking by a self-driving car acting upon a false-positive detection.
‘False-Negatives’ are most safety-critical
The occurrence of false-negatives is the most safety-critical aspect for vulnerable road users around a self-driving car. A false-negative occurs when a neural network determines that nothing is there when in fact something is actually there in the path of a self-driving car. The impact of this, i.e. a self-driving car not stopping or not slowing down when it should do so, has already been seen in context of industry-shaking crashes in which people have lost their lives.
Bringing it all together statistically – ‘mean Intersection over Union’ (mIoU)
The above concepts are brought together in a simple statistical framework.
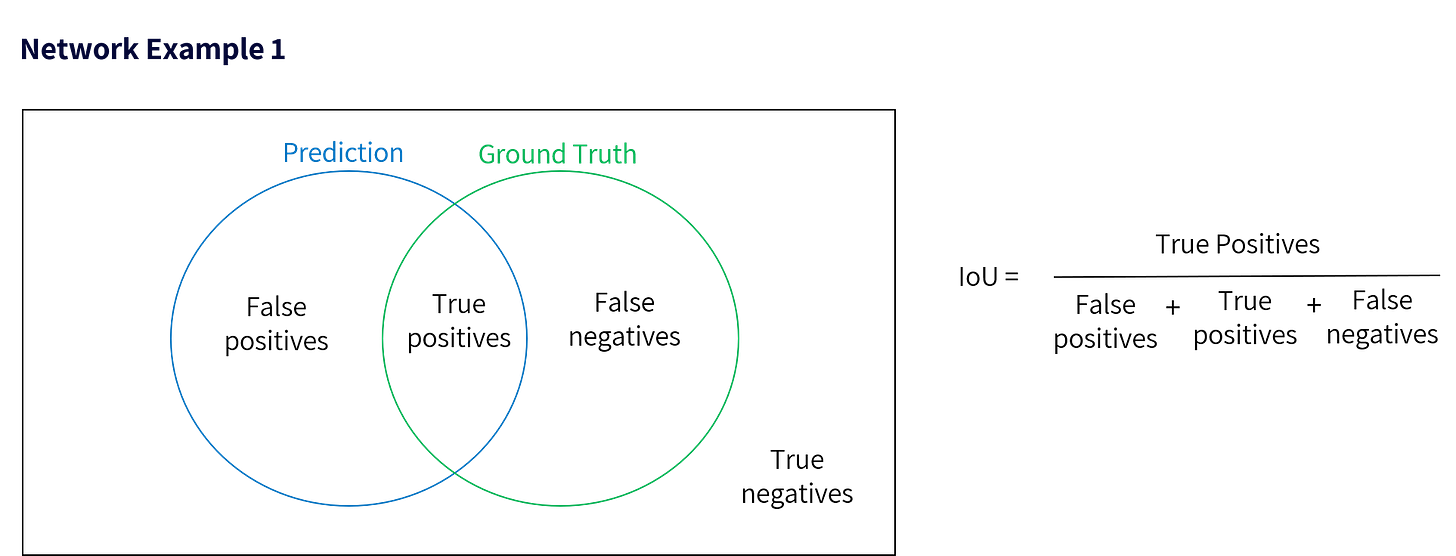
‘Intersection over Union’ (IoU) can be computed as the ‘area of intersection’ divided by the ‘area of union’. A mean value of the IoU over various observations generates a ‘mean Intersection over Union’ which is a metric used for determining the operational performance of neural networks. In the Network Example 1 shown above, the IoU of this network would be very low and regulators would not permit nor would insurers underwrite the risk of a self-driving car that has such a network deployed upon it for performing the perception task.
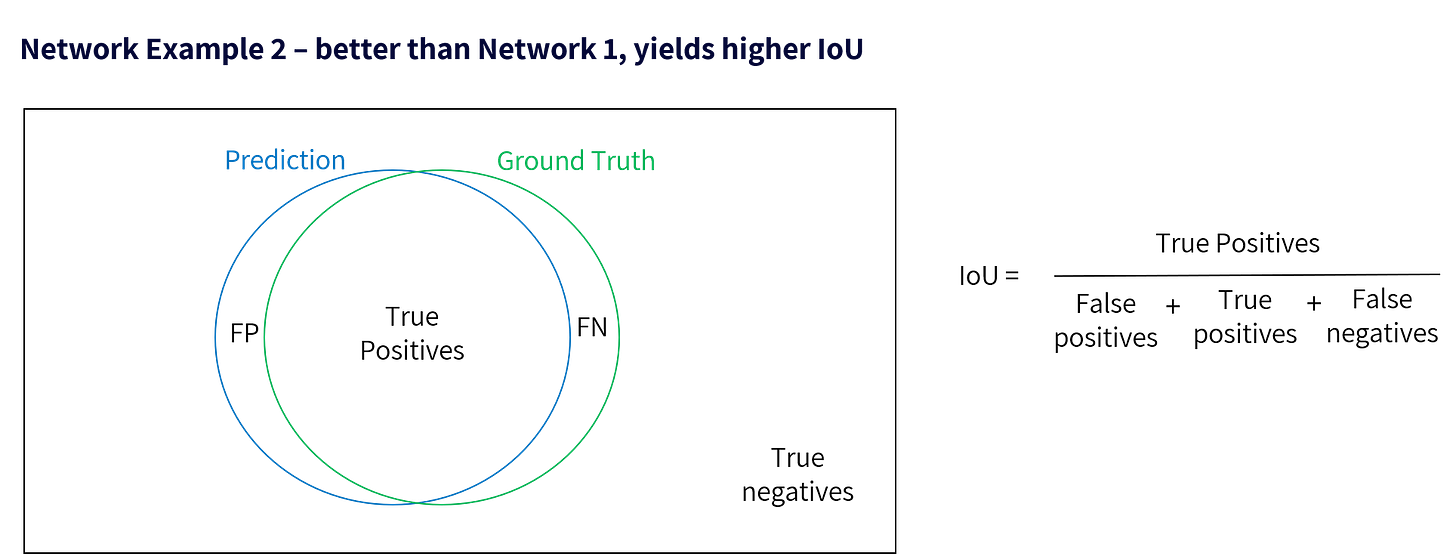
Network Example 2 above, shows a clear improvement over the performance of Network Example 1, as this neural network accounts for more of the true positives and suffers fewer false-positives and false-negatives.
A very good neural network (though still not theoretically ideal), would perform the perception function as shown in the Network Example 3 below and would theoretically generate an mIoU of close to 1, even on previously unseen data.
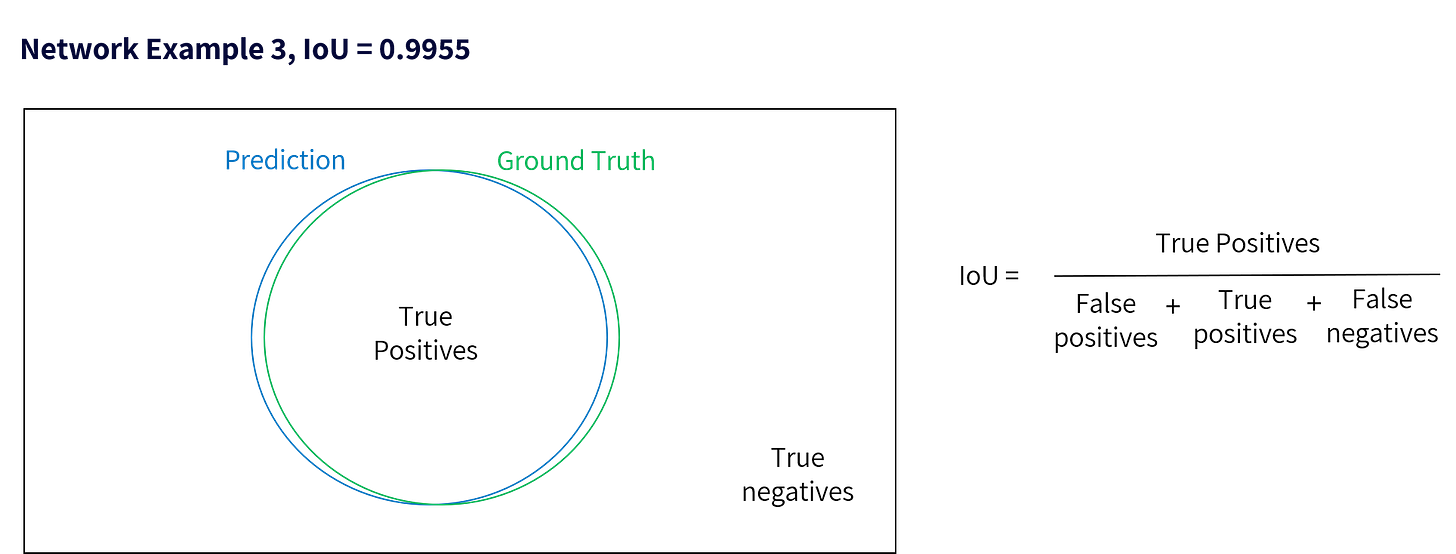
What is the ‘long-tail’ of corner cases?
Corner cases comprise those instances when a neural network encounters false-negatives and false-positives. It is therefore important to know that the susceptibility of a neural network to corner cases can be inferred from how far away its mIoU score is from a perfect score of 1 (100%), when operating on a vast quantum of previously unseen data.
mIoU - Known Unknown for Regulators
Self-driving car companies and ADAS providers may employ internal statistical assessments for measuring the performance level of their neural networks for perception, yet regulators are not made aware of any technology provider’s mIoU metrics. Nevertheless, conversations with regulators on this topic quickly reveal how some of them have readily accepted the claims of self-driving car companies and of ADAS providers, on the basis of simply having faith in their claims. Whereas technology suppliers would naturally be motivated to claim that their networks are generating performance levels close to Network Example 3. Perhaps, as the industry and the technology advances, technology developers will themselves make the effort to introduce greater transparency into making their perception technology capabilities much more scrutable in the interest of public safety.
In many currently deployed and commercially available automated driving technologies the perception performance of neural networks is actually closer to what is shown in Network Example 1 and Network Example 2, rather than Network Example 3 that most would like to claim.
At an industry-wide level, for most if not all automated driving or ADAS systems – Perception is certainly not solved.
Author: Zain Khawaja